Testing AI in the Melanoma: From Promise to Action
By Cody Barnett, MPH, MRA Senior Director of Communications & Patient Engagement | 8 March 2022 | Prevention, Science
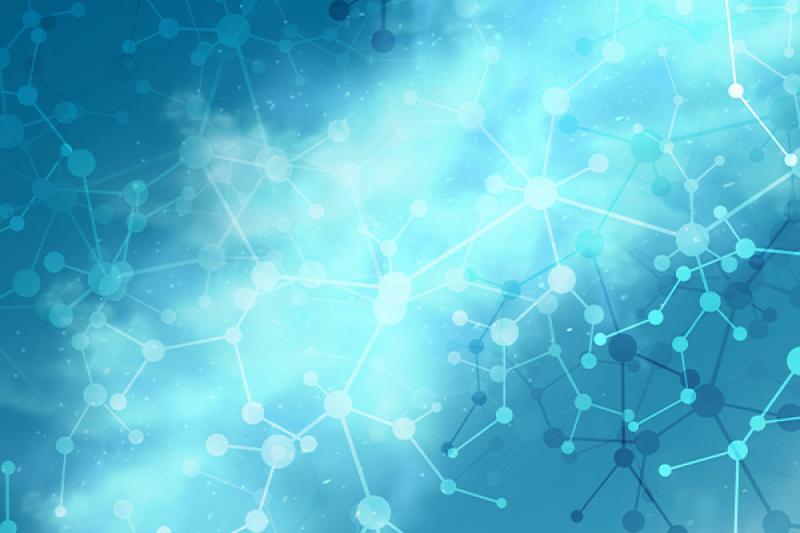
As recent history shows, when it comes to transformational melanoma research, innovation takes many forms. From treatment to detection — the field is evolving, and expanding rapidly to best meet the needs of people facing the disease. One researcher leading the way in melanoma detection is Dr. Roberto Novoa, a Clinical Associate Professor and Associate Program Director in the Division of Dermatopathology at Stanford University. Novoa is harnessing the power of artificial intelligence (AI) to more accurately detect and diagnose melanoma with a 2020 MRA Team Science Award supported by L’Oréal Dermatological Beauty Brands.
If you browse through your phone’s app store, you’ll find dozens of offerings related to melanoma. Several apps promise to help determine if a mole you’ve been staring at is cancerous or not based on the power of AI. While a great goal, none of these apps have earned approval from the Food and Drug Administration (FDA). However, to do so, apps — and the algorithms that they rely on — will need to be rigorously tested in the real world and across diverse populations. This is exactly where Dr. Novoa’s research is aimed.
Dr. Novoa, both a practicing dermatologist and dermatopathologist, has always been interested in recognizing patterns and making the right diagnosis. In fact, it’s what initially drew him into dermatology and to later subspecialize in dermatopathology. “While I liked many different aspects of medicine, I liked putting all the pieces together to find out what is going on … Visual diagnosis, it isn’t everything, but it is a big part of the practice of dermatology,” says Dr. Novoa. “Dermatopathology is an even further distillation of the same aspects that drew me to dermatology. You become a resource for all other dermatologists as you incorporate visual data with all available clinical data to get to an answer. I love it.”
Between splitting his time seeing patients and interpreting slides, Dr. Novoa also leads significant research efforts. “I’m interested in applying new technologies to the process of diagnosis. It all started for me seven years ago when I heard of some of the work being done in AI where computer scientists were using the technology to classify dog breeds. If they can do that, this technology can diagnose skin cancers.”
This is important because when melanoma is diagnosed at its earliest stage, it’s curable. However, significant disparities in the appropriate diagnosis of melanoma exist in the United States — especially for communities of color, economically disadvantaged individuals, and those living in rural areas.
While many studies have demonstrated that AI and machine learning can work to diagnose melanoma — at least in retrospective studies — real world assessment and practical lessons learned are still critically needed. What we don’t know is how these new technologies work in the clinic, what the ideal use-cases might be, what refinements are needed, and if any unforeseen benefits or pitfalls might exist.
In his study, Dr. Novoa and his team will evaluate the impact of their algorithm as a telemedicine triage tool within the Stanford Medical Center referral system. The team will then measure the efficacy of human-plus-machine performance versus either one alone. “Too often, data sets that are collected don’t represent the true complexity of pigmented lesions we see in the real world. In our study, we are correcting for that by going beyond the classic textbook — because in the real world, these lesions don’t read the textbook.”
His team is also collecting expansive imagery for each lesion studied, including multiple clinical pictures of the region of interest as well as dermatoscopic images. This combination is not frequently found in existing datasets, and Dr. Novoa is hopeful that combining both will improve diagnostic accuracy.
“We are trying to collect a broad variety of skin types in our analysis. This includes a partnership with Dr. Brian Gastman at the Cleveland Clinic, and the diversity of the patient population that institution serves. There are certainly issues about representation in the literature and datasets currently in use,” says Dr. Novoa. “We want to see how well our algorithm performs across all skin types and to make sure that the tools that we develop are equitable and applicable to all people.”
For Novoa — and other AI researchers — representative datasets and imagery are only part of the puzzle. That’s because for AI to work, a complex series of algorithms also need to be created. Taken together, these systems form a “neural network” capable of identifying patterns, classifying data, and even learning over time. Unfortunately, the inner workings and decisions made by neural networks aren’t easy to understand; this is often referred to as the “black box” of AI.
This lack of transparency reduces the utility of AI as a diagnostic companion for doctors and makes it harder to further improve the way the AI systems work. Novoa, and his team, are also taking steps to break this down — creating better insights and more opportunities to improve the technology over time.
“We wanted to see if we could move this technology beyond ‘the promise’ and see how it can help patients in day-to-day practice,” says Dr. Novoa. “We know there is potential, but now we want to know what are the best uses of artificial intelligence, and how can it help patients and doctors in real life?”